INTRODUCTION
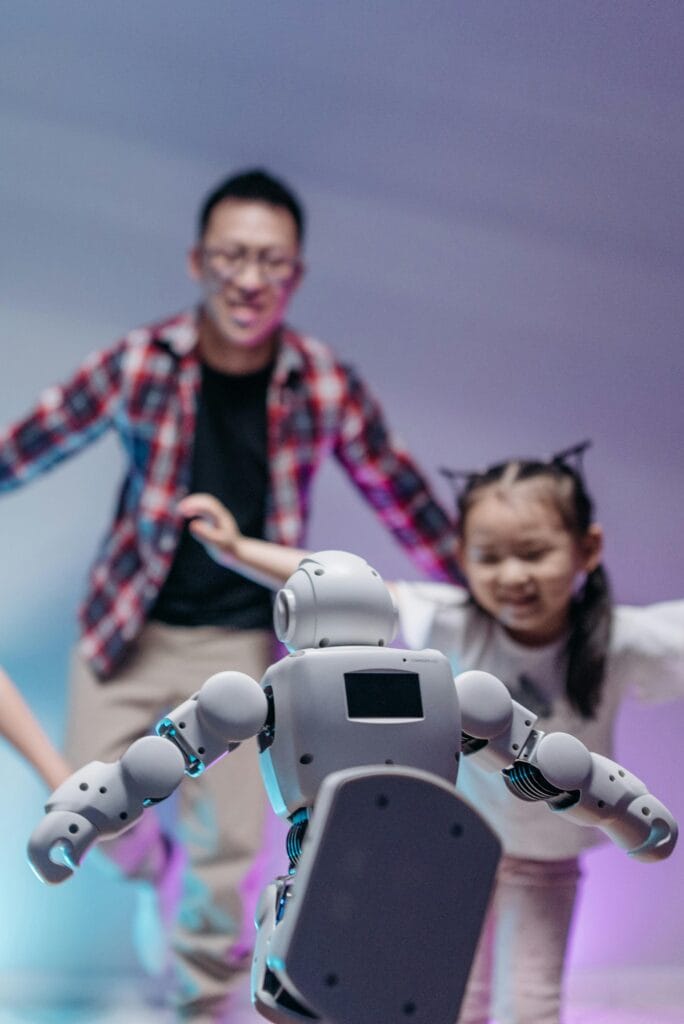
Electronic thinking, or ML, is man-made intelligent capability, a constituent of man-made insightful capability, which enlightens the methodology for creating assessments and models that could learn from information and hone their displays over time. It has emerged to be a very important feature of man-gained data ground, which gets machines involved in endeavors that were thought to be purely human undertakings like perceiving plans, producing ideas, and managing fantastic problems.
Algorithmic reasoning
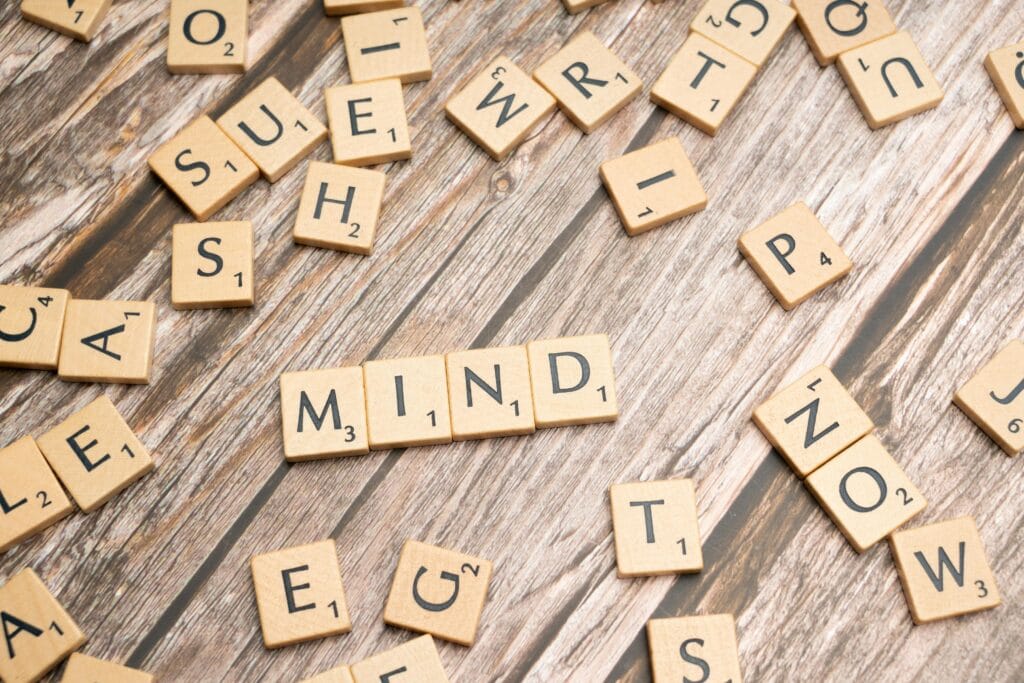
Computer-driven data structured computations for massive datasets to analyze patterns, connections, as well as relationships. These computations can then provide support to hypotheses or conclusions considering new, latent data. There are two fundamental categories of electronic reasoning:
Managed learning: In worked with learning, the estimate is prepared on a validated dataset where each data point is matched to the appropriate output. Evaluation solves an efficient means of assigning duties to outputs and can then begin making predictions on new, unlabeled data.
Free learning: In the execution learning, the estimation is based on an unlabeled dataset, where the server ranches are not related to a particular output. The estimation constructs a practical procedure for viewing models and patterns in the data with essentially no external course.
Key Strategies in man-made information
Direct apostatize: It is used for predicating quantitative characteristics, for example, house expenses or stock expenses.
Chosen break confidence: Applied for expecting matched results, for example, whether a client will mix.
Decision trees: Applied for seeking after decisions contemplating an improvement of rules.
Unpredictable backwoods: A social event method that joins different decision trees to support accuracy moreover.
Support vector machines (SVMs): Applied for get-together and apostatize endeavors.
Cortex connections: Inhaled by the human brain, mind networks are found that employed layered associated cortex hub neurons and can learn complicated models.
Implicit learning: Kind of cortex networks that use layers to glean complex features from data.
Applications of computer thinking in PC based data Improvement
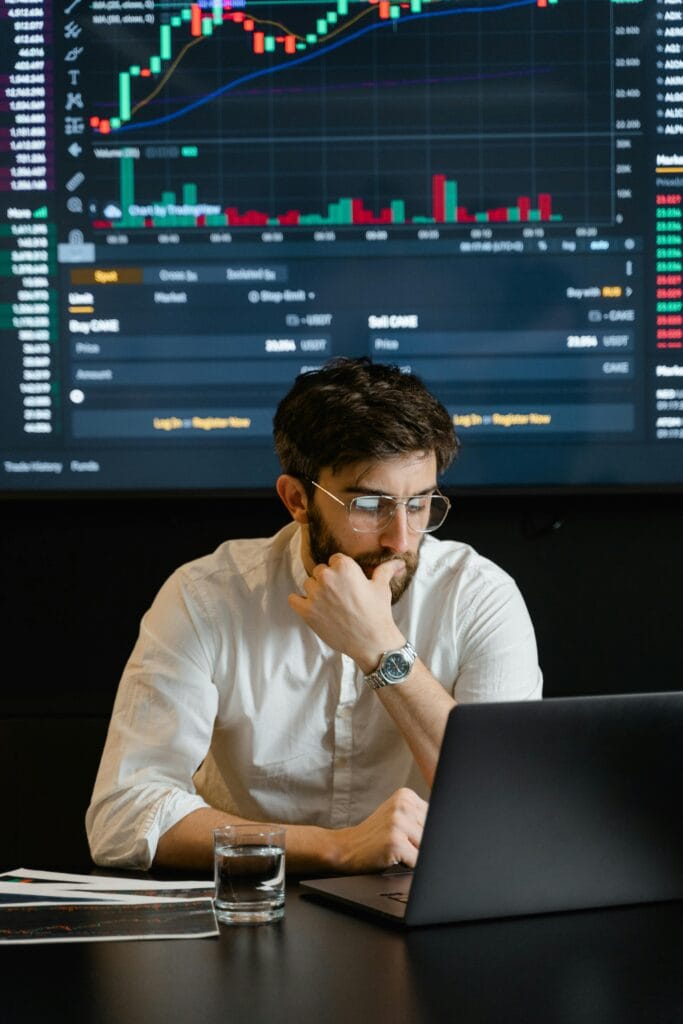
Artificial intelligence has many applications in intelligent thinking improvement, such as:
Current conventional language making due (NLP): artificial data are used to train models that can interpret and produce human language like chatbots, language understanding and also an evaluation.
Computer vision: PC based data used in training computer vision models by showing them images and files and letting them perceive and interpret objects and files.
Recommendation systems: artificial intelligence is applied in generating thought networks that can suggest entities, organizations, or content to customers based on their behaviors and guiding
Consensual judgement: artificial intelligence is applied in forecasting future events, such as customer pulse, pollution emissions, or financial market plans
Deception argument: artificial intelligence is applied in the identification of false implementations, such as credit card terrorism and security intimidation
Loads and Assessments in artificial intelligence
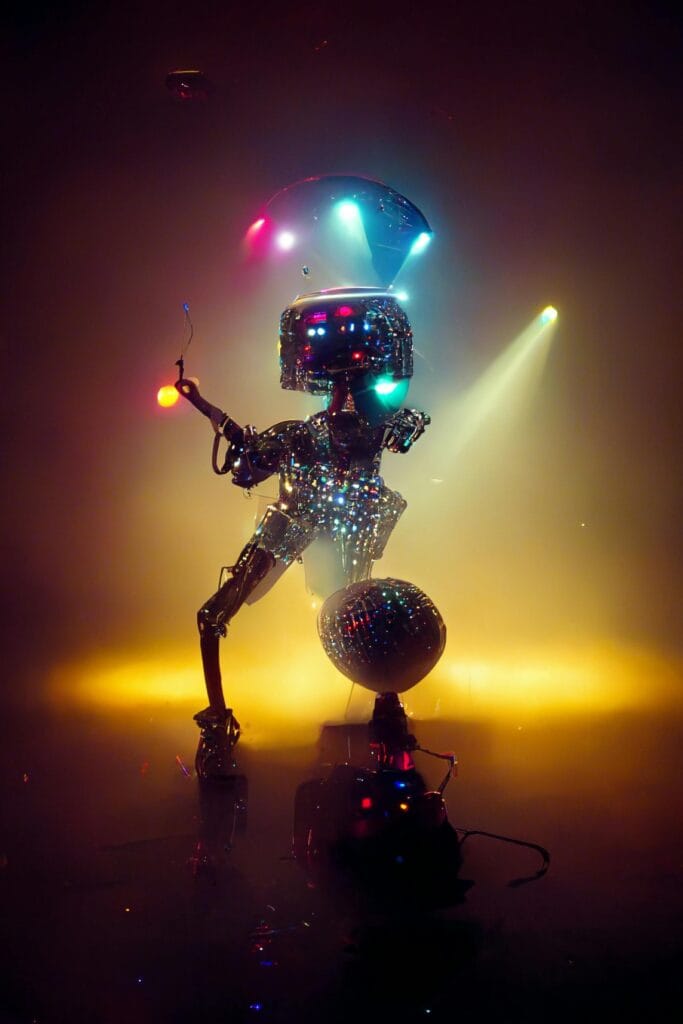
Data quality: The probability that data used in designing artificial intelligence models can be critical to accuracy and performance. If data quality is poor, then there’s very good possibility of biased and noisy output.
Overfitting: One of the biggest problems with any model is overfitting. The model is too rough while fitting the training data in very enthusiastic manner and thus causes terrible generalization on new, hidden data.
Interpretable: some artificial information models, for instance, enormous brain connections, are unintelligible to decode and hence challenging to know how they come up with their outputs.
Biased: artificial awareness models are prone to being biased if taken data is not necessarily representative of all them. This may lead to both unjustified or startling results.
Ethical concerns: computer-based information raise ethical considerations relating to data privacy, workmanship origin, and misuse.
Human-made consciousness in revised data progress is clean, and with it, the capability to set off so many disparate endeavors and influence our lives in absolutely enormous ways. As their rebuilds of data movement continue to provoke, we are bound to live more imaginative causes behind man-made consciousness that will rearrange the way we live and operate.
More Adventures
Solid re-enacted information (XAI):
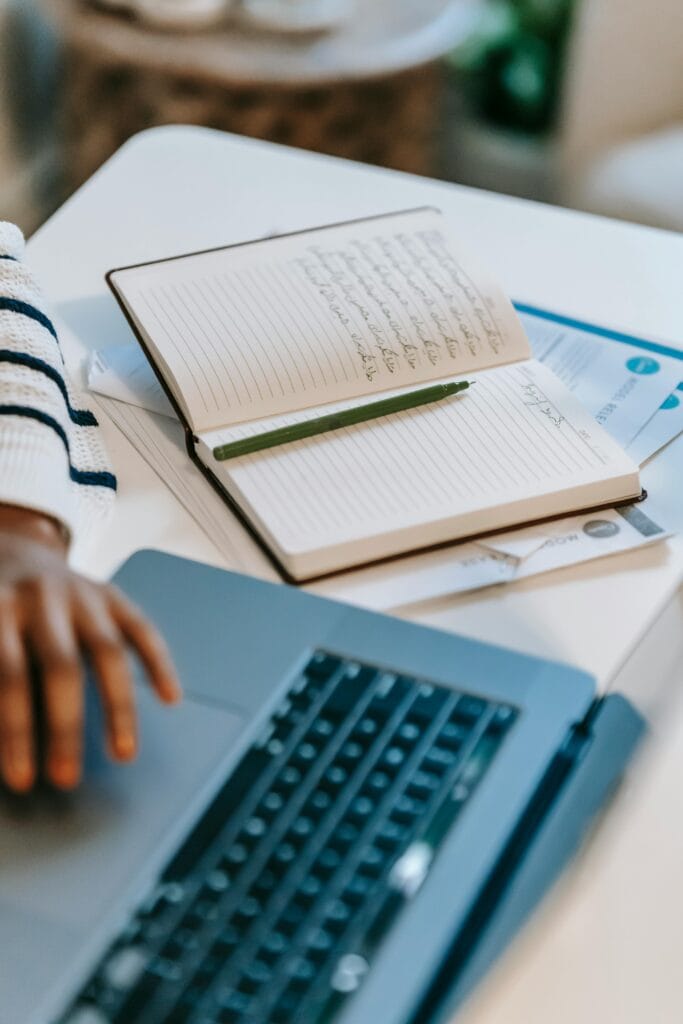
– XAI is another field of creating that astonishing lights on creating man-made considerations which are more interpretable, making it significantly more reason room wherein they to appear at their decisions. This is particularly fundamental in applications where straightforwardness and responsibility are pressing, as clinical benefits and cash.
Join Learning: This is a framework that will allow other parties to contribute to setting up a man-made understanding model without sharing their data. This will be very critical in providing data attestation and security, especially where the data used in the application is very sensitive.
AutoML: AutoML is a collection of techniques that modernize the best approach ever for controlling building and designing artificial information models. It can help experts in data as well as specialists to become much more critical while twirling around tasks at much higher level ends.
Generative models: Those are some sort of PC based data model which can generate new data. For example, pictures or text or music, etc. Generative models have incalculable potential applications, from workmanship and diversion to quiet disclosure and materials science.
Conclusion
AI is an incredible asset that can possibly open the maximum capacity of simulated intelligence. By creating calculations that can gain from information and work on their presentation after some time, we can make shrewd frameworks that can take care of perplexing issues, go with informed choices, and improve our lives in endless ways. As AI innovation keeps on advancing, we can hope to see significantly more imaginative applications that will shape the eventual fate of simulated intelligence.
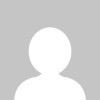