INTRODUCTION
Man-made consciousness (computer based intelligence) has quickly turned into an essential piece of our general public, affecting different enterprises from medical services to back. In any case, the rising predominance of artificial intelligence frameworks has additionally raised worries about predisposition. Predisposition in artificial intelligence frameworks can prompt prejudicial results, building up existing disparities and subverting public trust. To guarantee the moral and impartial sending of artificial intelligence, executing viable predisposition alleviation strategies is essential. This article will investigate the idea of predisposition in simulated intelligence, its likely results, and different methods for moderating predisposition in simulated intelligence frameworks.
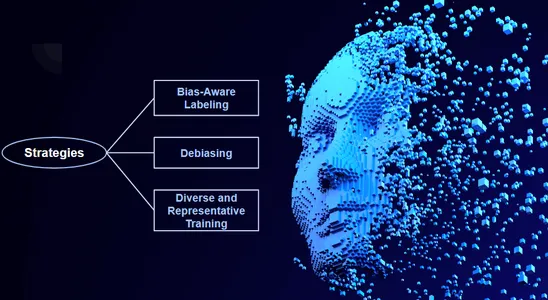
Figuring out Predisposition in computer based intelligence
Predisposition in artificial intelligence alludes to the propensity of computer based intelligence frameworks to display oppressive or uncalled for conduct towards specific gatherings. This predisposition can emerge from different variables, including:
One-sided Information: Assuming the information used to prepare simulated intelligence models is one-sided, the models will probably learn and propagate those inclinations. For instance, assuming a facial acknowledgment framework is prepared on a dataset that fundamentally incorporates pictures of white people, it might battle to precisely distinguish people with more obscure complexions.
Algorithmic Predisposition: The actual calculations can be one-sided, either because of configuration defects or unseen side-effects. For example, a few calculations may unintentionally enhance existing predispositions in the information.
Cultural Predisposition: man-made intelligence frameworks can reflect and support cultural predispositions that exist in reality. This can happen when simulated intelligence is utilized to go with choices that effect individuals’ lives, for example, employing or loaning.
Results of Predisposition in artificial intelligence
The results of predisposition in computer based intelligence can be extreme, both for people and society overall. A few potential results include:
Segregation: One-sided man-made intelligence frameworks can prompt oppression minimized gatherings, like racial minorities, ladies, and individuals with incapacities. This can altogether affect their lives, including their work amazing open doors, training, and admittance to medical care.
Loss of Trust: Predisposition in simulated intelligence can disintegrate public confidence in innovation and establishments. At the point when individuals see simulated intelligence frameworks as uncalled for or oppressive, they might be less inclined to take on or use them.
Support of Disparities: One-sided simulated intelligence frameworks can build up existing cultural imbalances by propagating generalizations and separation. This can intensify social and monetary incongruities.
Predisposition Moderation Strategies
To resolve the issue of predisposition in simulated intelligence, scientists and specialists have fostered various methods. These procedures can be extensively sorted into three principal regions:
- Information Driven Approaches
Information Quality: Guarantee that the information used to prepare man-made intelligence models is top notch, different, and delegate of the objective populace.
Information Expansion: Increment the variety of the preparation information by misleadingly producing new data of interest.
Predisposition Identification: Use procedures to recognize and address predispositions in the information prior to preparing the model.
- Calculation Driven Approaches
Decency Imperatives: Integrate reasonableness requirements into the artificial intelligence model’s goal capability to decently guarantee that the model treats various gatherings.
Predisposition Discovery and Rectification: Foster calculations that can distinguish and address predispositions in the model’s expectations.
Reasonable artificial intelligence: Make simulated intelligence models more straightforward and justifiable to people, which can assist with recognizing and address predispositions.
- Human-Driven Approaches
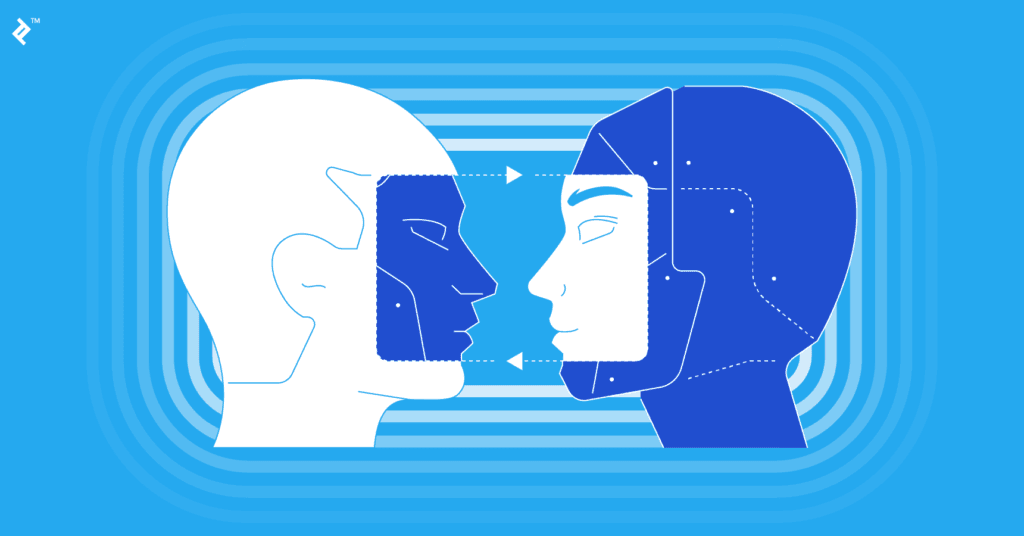
Variety and Incorporation: Guarantee that the groups creating and sending man-made intelligence frameworks are different and comprehensive, addressing various viewpoints.
Moral Rules: Create and comply to moral rules for the utilization of simulated intelligence, including standards of reasonableness, responsibility, and straightforwardness.
Public Commitment: Draw in with the general population to accumulate criticism and guarantee that simulated intelligence frameworks are created and sent in a manner that is predictable with cultural qualities.
Contextual investigations
To delineate the utilization of inclination moderation strategies, the following are a couple of contextual investigations:
Facial Acknowledgment: Numerous facial acknowledgment frameworks have been demonstrated to be one-sided against minorities. To resolve this issue, analysts have created procedures, for example, information expansion and reasonableness imperatives to work on the exactness of these frameworks for all people.
Recruiting Calculations: Some employing calculations have been found to victimize ladies and minorities. To alleviate this predisposition, organizations have executed measures, for example, eliminating orientation and other possibly oppressive data from resumes and involving decency limitations in the calculations.
Medical care computer based intelligence: computer based intelligence is progressively being utilized in medical care, yet there is a gamble that it might propagate existing wellbeing variations. To resolve this issue, specialists are creating simulated intelligence frameworks that are prepared on assorted datasets and consolidate decency imperatives to guarantee that they give fair consideration to all patients.
- Ongoing Monitoring and Observation
Trend Reports: Direct stable trend reports to observe and react to any emerging trends in PC-based information systems.
Performance Evaluations: Utilize authentic performance evaluations that are responsive to trend, for example, block integrity or equivalent gateway.
- Proper Systems
PC-based understanding Association: Establish and enforces liberal administrative structures for PC-based information, including rules for trend relief and participation.
In simple words Developed effort: Steered basic support to scale by and large measures for duplicated information ethics and management.
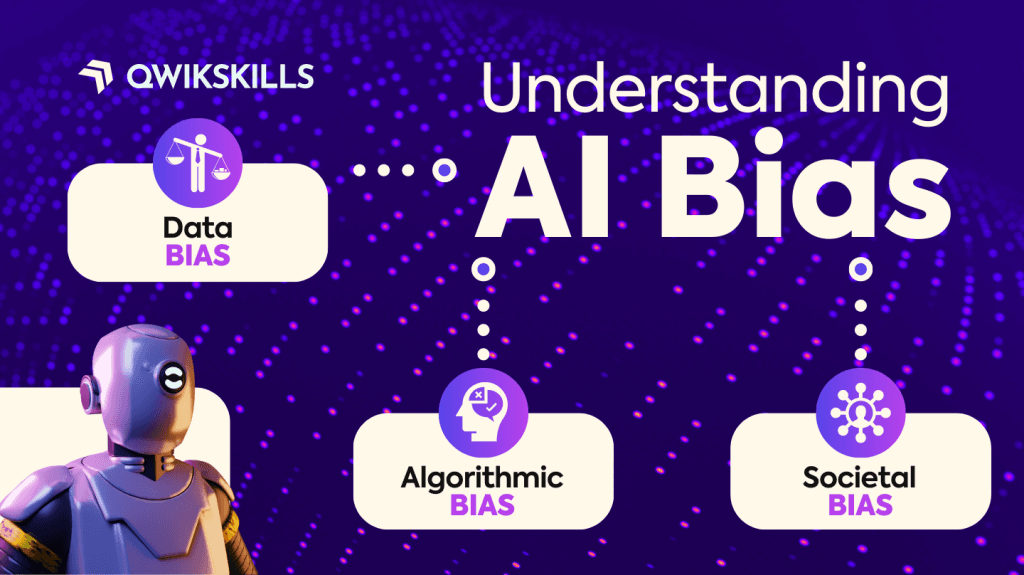
- Public Trust and Transparency
Reasonable artificial intuition: Make PC based information systems less hard to understand and clear to the public so it could create a feeling of trust and remove fear and apprehension of bias.
Public Involvement : Involve all the people in the new building and design of revived information architectures so their characteristics and desires are recognized.
- Ethical Issues
Structural openness: Computer-based knowledge applications do not deceive, which falls within fundamental feasibility rules such as the freedom from discrimination and to privacy.
Social justice: Highly formalized and money-spinning effects of computer-based knowledge models are seen as attempts to mitigate harmful effects.
- Latest Trends
Generative computer-based knowledge: Generative artificial knowledge models, such as language models with hypothesis enforcement, could be prejudiced in all sorts of ways.
Man-handle with cerebral capacity in High-Stakes Decisions: Tread with caution while injecting PC-based information to high-stakes decisions, such as policing clinical benefits, and also ensure human control is available.
Conclusion
Tendency balance in simulated data is a wonderful and continuous test. By sagaciously considering the issues arisen from this article and developing a way proactive approaches to watch out for them, we can be sure that PC based information structures are developed and disseminated in a manner that is moral, fair, and useful to society. This will require a supportive effort from learned authorities, specialists, policymakers, and general society.
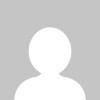