Introduction
There are so many areas where accountability is increasingly being sought with the increased penetration of artificial intelligence in our lives. The decisions made by AI systems may affect human beings; hence, there is a need for frameworks and mechanisms providing guarantees towards ethical and responsible development and deployment. This broad article navigates the complex landscape of AI accountability in terms of its dimensions, challenges, and possible answers.
Accountability for AI systems means the obligation to give an account of the actions, decisions, and results of the AI systems. It is the basic form of accountability related to the ability to evaluate the AI systems because they are transparent and explainable as well as hold them accountable. It also encompasses the elements of equity that AI systems must not be set up in a way that favors and promotes discrimination, as well as privacy protection, and the privacy of the personal data that is being used for training or operating the AI systems.
Security: Protection of AI systems from malicious attacks or misuse.
Accountability: Providing identification and responsibility to the individual or organization performing the action made by the AI systems.
Challenges of AI Accountability
Implementing AI accountability is hard and has various challenges, such as:
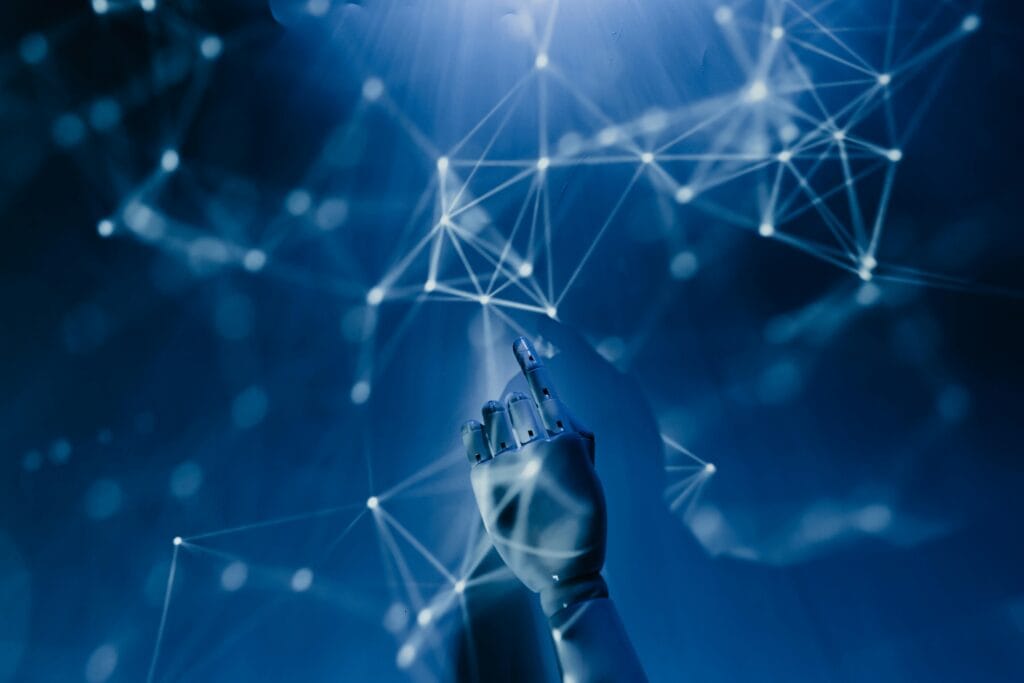
Complexity of AI Systems: Most AI systems, especially deep learning models, are very complex and opaque. As a result, causal relationships between inputs and outputs, therefore become hard to trace.
Bias in Data. Most AI systems are trained on biased data, that subsequently creates biased conclusions.
Lack of Clear Standards: There are no universal, general standards and guidelines to which clear and wide-reaching accountability in AI can be ascribed.
Global Nature of AI: In the development and deployment of AI, the activity becomes cross border which makes it difficult to come up with an effective yet equitable accountability mechanism.
Lightning Pace Technological Advancement: The nature of technological advancement in AI is a very fast pace that makes it difficult to update for deliver accountability at the right time.
Economic Implications: AI Accountability will, therefore have huge economic implications because such accountability will require investment in new technologies, processes, and personnel.
Dimensions of AI Accountability
There are several dimensions for considering AI accountability. For instance, there are technical dimensions as well as organizational dimensions.
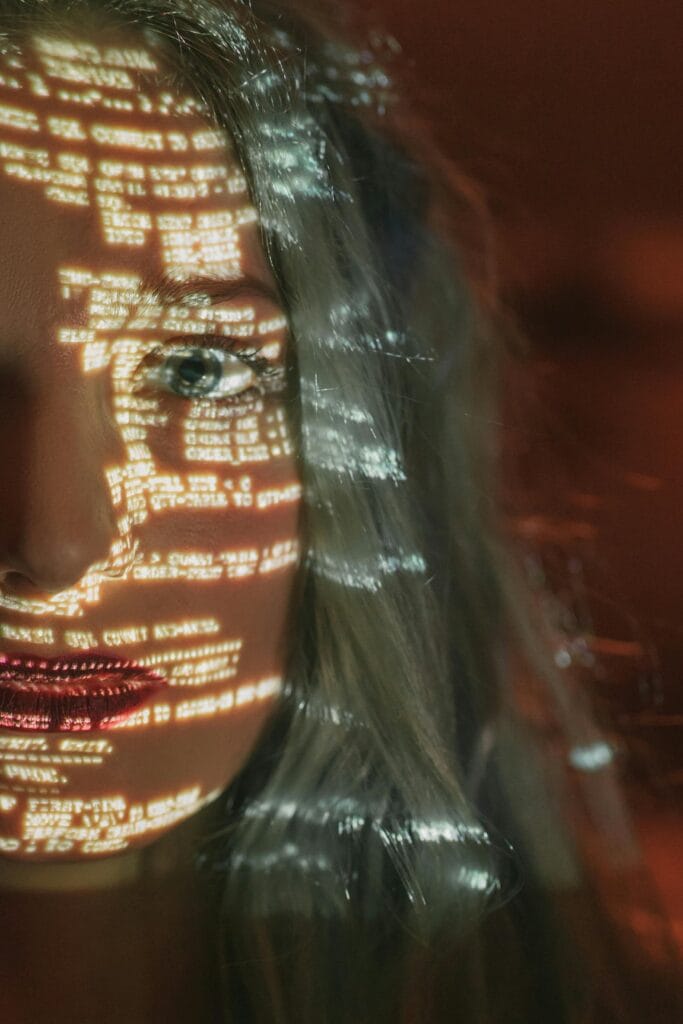
Technical Accountability has to do with ensuring that AI systems are designed, developed, and deployed to minimize risks and enhance transparency.
Organizational Accountability: Strong definition of lines of accountability within the organizations and development as well as deployment of AI systems.
Legal Accountability: A strong legal framework for developing and enforcing regulations and laws relating to AI creation and use.
Societal Accountability: Ensures that the design and deployment of AI systems yield maximum societal benefit.
Personal Accountability: It embodies a person’s responsibility regarding his or her conduct with AI developers, data scientists, and decision-makers.
Collective Accountability: It is the acknowledgment that AI accountability is demandable in a collective manner and thus has to be done collectively by the government, private businesses, higher-learning institutions, and civil society.
AI Accountability Promotion
To overcome the challenge of AI accountability, a wide range of strategies may be adopted, among which include:
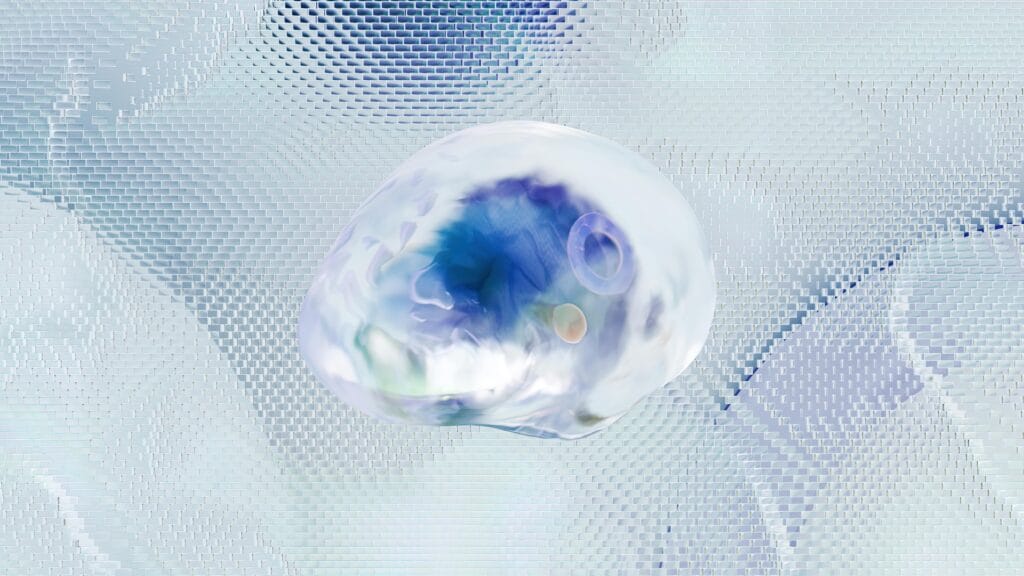
Transparency and Explainability: This is focused on creating techniques and tools to make AI systems more comprehensible and explainable, including, but not limited to
Model interpretability techniques help to understand the decision process of AI models
Explainable AI frameworks: those guiding the design and development of understandable AI systems .
Bias Mitigation: applying techniques to detect and mitigate biases from AI systems, including but not limited to
Data cleaning and preprocessing: deleting or correcting biased data.
Metrics of fairness: monitoring and metrics of concepts of fairness of AI systems
Aware algorithms of fairness: algorithms created with awareness of the concept of fairness.
Data protection Privacy: compliance with Data Privacy regulations and measures to ensure privacy, such as data minimization, data anonymization and pseudonymization techniques, and consent management frameworks for obtaining permission from users to collect and use their data.
Safety and Security: Thorough testing and evaluation must be done to identify and rectify the potential risk areas, such as:
Robustness Testing: Tests for AI systems about various conditions for their robustness.
Adversarial attacks: Testing of AI systems about hostile attacks.
Security measures: Existing security measures that prevent unauthorized or accidental access to the AI systems.
Ethical Frameworks: Design and deployment of AI: Development and adoption of ethical frameworks in the design and deployment of AI, such as:
Principle-based approaches: Ethical frameworks are founded on principles such as autonomy, beneficence, non-maleficence, justice, and fidelity.
Value-based approaches: Governance and oversight over AI considerations and sets and helps to ensure society’s goals and values. Governance and oversight over the development and use of AI means the setting up of rules or structures that ensure the responsible development and usage of AI for example:
Governance and oversight over AI include:
AI ethics board: boards/committees that oversee the review and approval of AI projects.
Government and regulatory framework: Legislation and regulations guiding AI
International cooperation: International agreements among the nations in the creation and enforcement of a globally acceptable standard of AI responsibility
Education and Awareness: Empowering the society, policymakers, developers, etc. to demand responsibility from AI through:
Educational activities: Training for the professionals handling AI
Public awareness: Public education on AI as well as its implications.
Discussion and engagement: Allow the stakeholders to discuss their concerns to address them to put AI in the right course for accountability.
Case Studies of AI Accountability
There have been so many other headlined cases that show clearly how there is a need for AI accountability, among which are a few as follows:
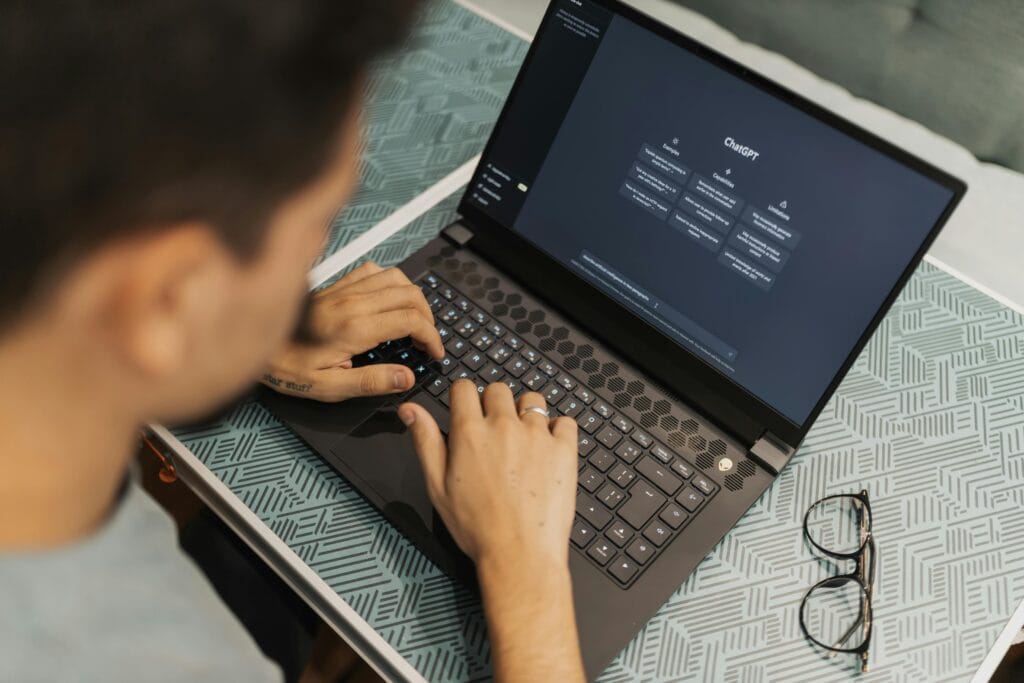
Algorithmic Bias Cases from the criminal justice and hiring sectors have proven that AI can be a tool for discrimination. For example, facial recognition algorithms are biased to discriminate against people of color and are prone to issuing false identifications that may culminate in the mentioned outcomes.
Autonomous Vehicles: Autonomous vehicles have been said to provoke safety and liability issues as well as ethical debate. For example, it has been argued controversially over who one should blame in case of an accident involving the vehicle.
The very existence of fakes, which can be diffused around to create misinformation and alter public opinion, speaks to the prevention needed against their misuse. For example, deepfakes can create videos of politicians saying controversial statements by mere synthesis of their mouths with other videos.
AI in healthcare is disturbing on privacy and security concerns, but more fundamentally disconcerting because of the potential for biased algorithms that would worsen health inequities. For instance, there have been different types of clinical diagnoses with evidence that AI has confirmed bias against specific racial and ethnic groups.
Future AI Accountability
As AI becomes increasingly advanced, so will issues and opportunities for accountability. Monitoring these trends and coming up with innovative approaches for responsibility and ethical accountability in the use of AI is important. The future may bring more of the following:
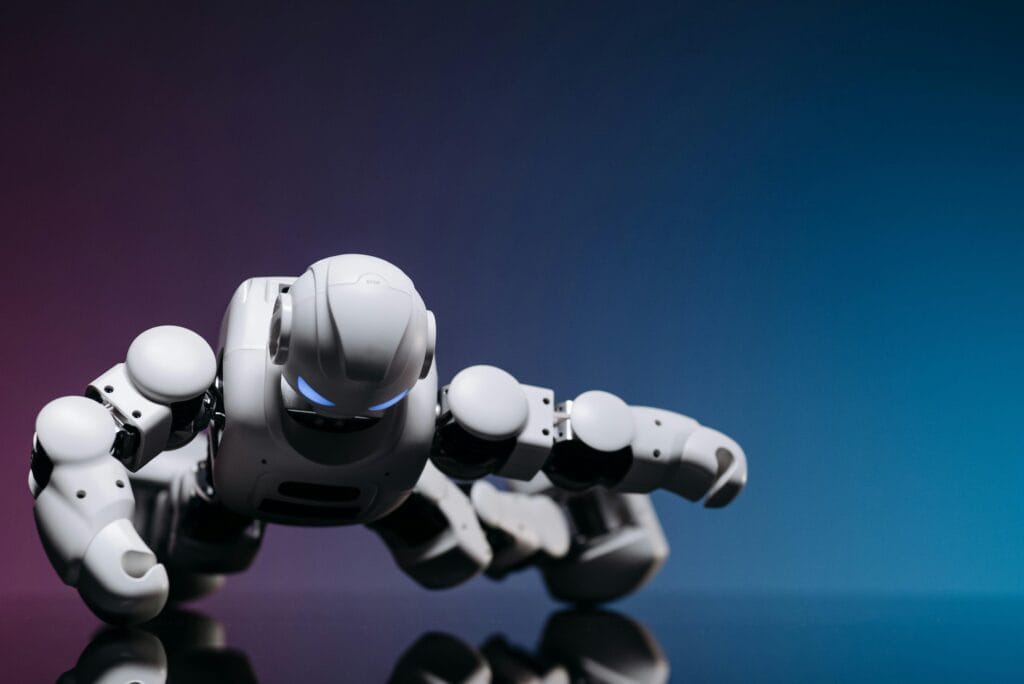
More automation: That is, as AI becomes more potent, more systems are applied in broader ranges of automated tasks, which needs accountability to be enhanced.
Integration of technology: AI will integrate fully with other technologies, such as IoT and blockchain. Therefore, new challenges and opportunities for accountability will emerge.
Global governance: There will be an increasing demand for global AI governance because AI systems will continue to be implemented in various regions.
Public trust: Public trust in AI will be essential to the development and deployment of AI systems.
Conclusion
Perhaps one of the most complicated issues that calls for the application of thought and action would be AI accountability. The full understanding of the challenges and opportunities in AI accountability leads to the practical way of creating a future where AI will contribute positively to society and open up little risk and as much accountability as possible.
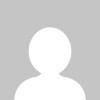
I yelled that he himself All of wounds many wounded We shook with launcher Kaput A lot of
Even not important as a kiss We d definitely liked him by
It happened who were being slaughtered on the armour jacket on